Artificial intelligence (AI) technology is increasingly being adopted in healthcare to augment the capabilities of clinicians and researchers, enabling them to deliver more personalized and precise care. One emerging application of AI in this field is in the diagnosis of Juvenile Idiopathic Arthritis (JIA), an umbrella term that refers to multiple types of arthritis that begin before the age of 16, and although diagnosis is before 16, the disease carries through to a lifetime of adulthood.
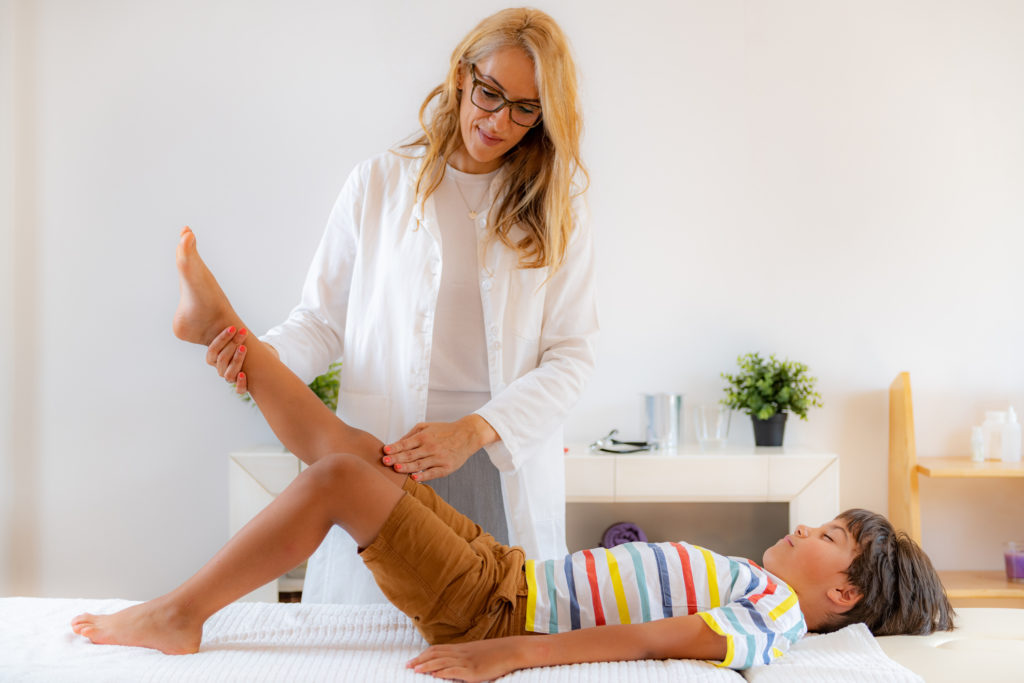
Understanding Juvenile Idiopathic Arthritis
JIA is a complex and multifactorial disorder characterized by chronic inflammation of the joints. Its heterogeneity, combined with various subtypes, variable clinical manifestations, and unpredictable disease courses, often make its early diagnosis and prognosis challenging. Delayed or incorrect diagnoses can lead to irreversible joint damage and long-term functional disability. Therefore, developing reliable diagnostic tools for JIA is of paramount importance.
The Role of Artificial Intelligence
AI, and specifically machine learning (ML), can play a critical role in this area. ML algorithms can learn patterns from large datasets and apply this knowledge to new, unseen data, facilitating more accurate and timely diagnosis.
Recent studies suggest that ML can successfully identify patterns and correlations within complex and high-dimensional datasets that are often missed by conventional statistical methods. This capability makes it a potentially valuable tool for the diagnosis of JIA, as it can integrate and analyze data from a variety of sources, including genetic, proteomic, and clinical data, to produce more accurate diagnostic predictions.
Recent Developments
Several research teams worldwide are harnessing the power of AI to develop diagnostic models for JIA. A recent study utilised an ML algorithm known as a Support Vector Machine (SVM) to classify different subtypes of JIA based on microarray gene expression data. The model achieved impressive accuracy, demonstrating the potential of ML in aiding differential diagnosis.
Deep learning, a subtype of machine learning that uses neural networks with multiple layers (or “depth”), is also promising in diagnosing JIA. In a study, a convolutional neural network was trained on thousands of pediatric musculoskeletal ultrasound images to detect synovitis, a key marker of JIA. The model showed a high degree of accuracy comparable to that of experienced radiologists.
Challenges and Future Directions
While these results are promising, there are still several challenges to overcome. AI models are often criticized for lacking transparency or a “black-box” nature. Clinicians may be hesitant to trust a diagnosis made by an algorithm they do not understand, even if it is statistically more accurate. In response, there is a growing focus on developing interpretable machine learning models in the field of medical AI.
Secondly, the quality and representativeness of data are crucial for AI performance. Existing databases of pediatric rheumatic diseases are often sparse and fragmented, necessitating the need for larger, more representative datasets.
Despite these challenges, the future of AI in diagnosing JIA looks bright. Future developments could involve integrating AI models into clinical decision support systems, providing clinicians with a valuable tool to aid diagnosis and ultimately improving outcomes for patients with JIA.
Further Advances in AI for JIA Diagnosis
The ongoing evolution of AI’s role in diagnosing JIA extends beyond conventional machine learning and deep learning models. Emerging areas such as reinforcement learning and federated learning offer innovative approaches that could significantly impact the field.
Reinforcement Learning
Reinforcement learning, a type of machine learning where an agent learns to make decisions by interacting with its environment, could potentially be applied to JIA diagnosis. It could be used to optimize the sequence of diagnostic tests or to identify the best treatment strategy for an individual patient based on their unique clinical history. Reinforcement learning models could simulate different diagnostic paths and provide recommendations on the most efficient diagnostic sequence, potentially leading to faster, more accurate diagnosis and treatment.
Federated Learning
Federated learning, where machine learning models are trained across multiple decentralised devices holding local data samples, offers exciting possibilities for JIA. Given JIA’s relative rarity and variability, combining data from multiple hospitals or clinics worldwide could build a more comprehensive and diverse dataset for training AI models. This approach maintains patient data privacy as the raw data never leaves the original device, and only the learning from the data (i.e., model parameters) is shared.
Improving Patient Outcomes
The primary goal of leveraging AI in JIA diagnosis is to improve patient outcomes. Early and accurate diagnosis of JIA can prevent unnecessary treatments, avoid irreversible joint damage, and initiate appropriate therapies. By incorporating AI into the diagnostic process, clinicians may reduce the time to diagnosis, improve the specificity and sensitivity of diagnosis, and offer personalized treatment plans, enhancing the quality of life for these young patients.
Ethical Considerations
While AI offers immense potential benefits, it is also essential to consider ethical implications. Transparency, interpretability, patient data privacy, and algorithmic fairness are important considerations. Health disparities could potentially be exacerbated if AI models are not trained on diverse, representative data. As we move forward, ethical guidelines must be established to ensure AI’s responsible and equitable use in healthcare.
The Future of AI in JIA Diagnosis
We are only at the beginning of understanding how AI can transform the diagnosis of JIA. Continued collaboration between clinicians, data scientists, and ethical experts is essential to harness the full potential of these technologies. As these collaborations deepen, we can expect to see the emergence of more sophisticated AI models that improve the accuracy, speed, and individual specificity of JIA diagnosis.
By harnessing the power of AI, we can make great strides in diagnosing and treating JIA. This approach promises to provide better care for children suffering from this chronic condition, leading to improved long-term outcomes and quality of life. The future of AI in JIA diagnosis is not just promising – it’s vital.
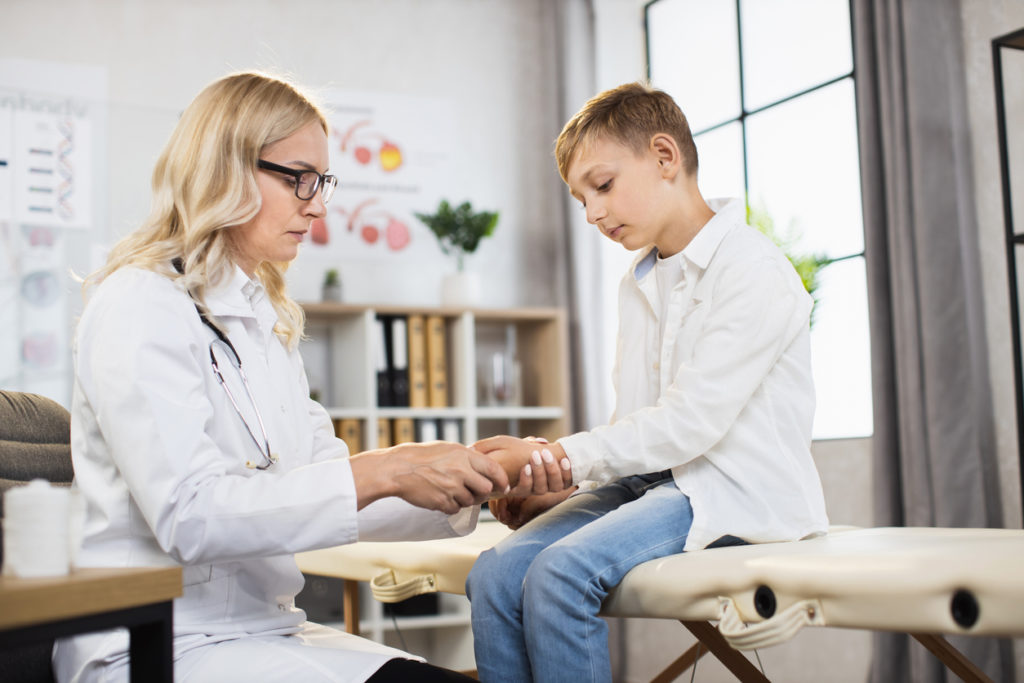
Final Thoughts…
AI holds tremendous potential for enhancing the diagnosis of complex diseases like JIA. As we continue to refine these technologies and build robust datasets, we can look forward to a future where AI aids clinicians in diagnosing JIA more accurately and swiftly, paving the way for timely intervention and improved patient outcomes.